Apache Flume is a distributed, reliable, and available system for efficiently collecting, aggregating and moving large amounts of log data from many different sources to a centralized data store. Flume is designed for high-volume ingestion into Hadoop of event-based data.
The use of Apache Flume is not only restricted to log data aggregation. Since data sources are customizable, Flume can be used to transport massive quantities of event data including but not limited to network traffic data, social-media-generated data, email messages and pretty much any data source possible. There are currently two release code lines available, versions 0.9.x and 1.x.
System Requirements
- Java Runtime Environment – Java 1.6 or later (Java 1.7 Recommended)
- Memory – Sufficient memory for configurations used by sources, channels or sinks
- Disk Space – Sufficient disk space for configurations used by channels or sinks
- Directory Permissions – Read/Write permissions for directories used by agent
Architecture
To use Flume, we need to run a Flume agent, which is a long-lived Java process that runs sources and sinks, connected by channels. A source in Flume produces events and delivers them to the channel, which stores the events until they are forwarded to the sink. You can think of the source-channel-sink combination as a basic Flume building block.
Data flow model – A Flume event is defined as a unit of data flow having a byte payload and an optional set of string attributes. A Flume agent is a (JVM) process that hosts the components through which events flow from an external source to the next destination (hop).
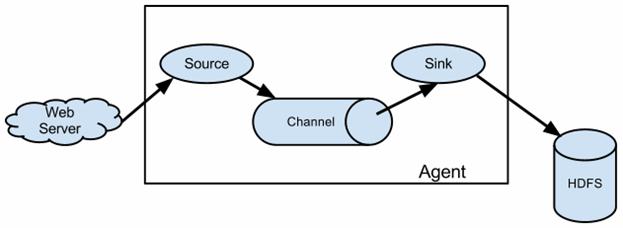
A Flume source consumes events delivered to it by an external source like a web server. The external source sends events to Flume in a format that is recognized by the target Flume source. For example, an Avro Flume source can be used to receive Avro events from Avro clients or other Flume agents in the flow that send events from an Avro sink. A similar flow can be defined using a Thrift Flume Source to receive events from a Thrift Sink or a Flume Thrift Rpc Client or Thrift clients written in any language generated from the Flume thrift protocol. When a Flume source receives an event, it stores it into one or more channels. The channel is a passive store that keeps the event until it’s consumed by a Flume sink. The file channel is one example – it is backed by the local filesystem. The sink removes the event from the channel and puts it into an external repository like HDFS (via Flume HDFS sink) or forwards it to the Flume source of the next Flume agent (next hop) in the flow. The source and sink within the given agent run asynchronously with the events staged in the channel.
Complex Flows – Flume allows a user to build multi-hop flows where events travel through multiple agents before reaching the final destination. It also allows fan-in and fan-out flows, contextual routing and backup routes (fail-over) for failed hops.
Reliability – The events are staged in a channel on each agent. The events are then delivered to the next agent or terminal repository (like HDFS) in the flow. The events are removed from a channel only after they are stored in the channel of next agent or in the terminal repository. This is a how the single-hop message delivery semantics in Flume provide end-to-end reliability of the flow.
Flume uses a transactional approach to guarantee the reliable delivery of the events. The sources and sinks encapsulate in a transaction the storage/retrieval, respectively, of the events placed in or provided by a transaction provided by the channel. This ensures that the set of events are reliably passed from point to point in the flow. In the case of a multi-hop flow, the sink from the previous hop and the source from the next hop both have their transactions running to ensure that the data is safely stored in the channel of the next hop.
Recoverability – The events are staged in the channel, which manages recovery from failure. Flume supports a durable file channel which is backed by the local file system. There’s also a memory channel which simply stores the events in an in-memory queue, which is faster but any events still left in the memory channel when an agent process dies can’t be recovered.
Flume uses separate transactions to guarantee delivery from the source to the channel and from the channel to the sink. In the example in the previous section, the spooling directory source creates an event for each line in the file. The source will only mark the file as completed once the transactions encapsulating the delivery of the events to the channel have been successfully committed.
Similarly, a transaction is used for the delivery of the events from the channel to the sink. If for some unlikely reason the events could not be logged, the transaction would be rolled back and the events would remain in the channel for later redelivery.
The channel we are using is a file channel, which has the property of being durable: once an event has been written to the channel, it will not be lost, even if the agent restarts. (Flume also provides a memory channel that does not have this property, since events are stored in memory. With this channel, events are lost if the agent restarts. Depending on the application, this might be acceptable. The trade-off is that the memory channel has higher throughput than the file channel.)
Large datasets are often organized into partitions, so that processing can be restricted to particular partitions if only a subset of the data is being queried. For Flume event data, it’s very common to partition by time. A process can be run periodically that transforms completed partitions (to remove duplicate events, for example).
It’s normally a good idea to use a binary format for storing your data in, since the resulting files are smaller than they would be if you used text. For the HDFS sink, the file format used is controlled using hdfs.fileType and a combination of a few other properties.
Fan out is the term for delivering events from one source to multiple channels, so they reach multiple sinks. A sink group allows multiple sinks to be treated as one, for failover or load-balancing purposes. If a second-tier agent is unavailable, then events will be delivered to another second-tier agent and on to HDFS without disruption.
Setup
A Flume installation is made up of a collection of connected agents running in a distributed topology. Agents on the edge of the system (co-located on web server machines, for example) collect data and forward it to agents that are responsible for aggregating and then storing the data in its final destination. Agents are configured to run a collection of particular sources and sinks, so using Flume is mainly a configuration exercise in wiring the pieces together.
Setting up an agent – Flume agent configuration is stored in a local configuration file. This is a text file that follows the Java properties file format. Configurations for one or more agents can be specified in the same configuration file. The configuration file includes properties of each source, sink and channel in an agent and how they are wired together to form data flows.
Configuring individual components – Each component (source, sink or channel) in the flow has a name, type, and set of properties that are specific to the type and instantiation. For example, an Avro source needs a hostname (or IP address) and a port number to receive data from. A memory channel can have max queue size (“capacity”), and an HDFS sink needs to know the file system URI, path to create files, frequency of file rotation (“hdfs.rollInterval”) etc. All such attributes of a component needs to be set in the properties file of the hosting Flume agent.
Wiring the pieces together – The agent needs to know what individual components to load and how they are connected in order to constitute the flow. This is done by listing the names of each of the sources, sinks and channels in the agent, and then specifying the connecting channel for each sink and source. For example, an agent flows events from an Avro source called avroWeb to HDFS sink hdfs-cluster1 via a file channel called file-channel. The configuration file will contain names of these components and file-channel as a shared channel for both avroWeb source and hdfs-cluster1 sink.
Starting an agent
An agent is started using a shell script called flume-ng which is located in the bin directory of the Flume distribution. You need to specify the agent name, the config directory, and the config file on the command line:
$ bin/flume-ng agent -n $agent_name -c conf -f conf/flume-conf.properties.template
Now the agent will start running source and sinks configured in the given properties file.
A simple example – Here, we give an example configuration file, describing a single-node Flume deployment. This configuration lets a user generate events and subsequently logs them to the console.
# example.conf: A single-node Flume configuration
# Name the components on this agent
a1.sources = r1
a1.sinks = k1
a1.channels = c1
# Describe/configure the source
a1.sources.r1.type = netcat
a1.sources.r1.bind = localhost
a1.sources.r1.port = 44444
# Describe the sink
a1.sinks.k1.type = logger
# Use a channel which buffers events in memory
a1.channels.c1.type = memory
a1.channels.c1.capacity = 1000
a1.channels.c1.transactionCapacity = 100
# Bind the source and sink to the channel
a1.sources.r1.channels = c1
a1.sinks.k1.channel = c1
This configuration defines a single agent named a1. a1 has a source that listens for data on port 44444, a channel that buffers event data in memory, and a sink that logs event data to the console. The configuration file names the various components, then describes their types and configuration parameters. A given configuration file might define several named agents; when a given Flume process is launched a flag is passed telling it which named agent to manifest.
Given this configuration file, we can start Flume as follows:
$ bin/flume-ng agent –conf conf –conf-file example.conf –name a1 -Dflume.root.logger=INFO,console
Note that in a full deployment we would typically include one more option: –conf=<conf-dir>. The <conf-dir> directory would include a shell script flume-env.sh and potentially a log4j properties file. In this example, we pass a Java option to force Flume to log to the console and we go without a custom environment script.
From a separate terminal, we can then telnet port 44444 and send Flume an event:
$ telnet localhost 44444
Trying 127.0.0.1…
Connected to localhost.localdomain (127.0.0.1).
Escape character is ‘^]’.
Hello world! <ENTER>
OK
The original Flume terminal will output the event in a log message.
12/06/19 15:32:19 INFO source.NetcatSource: Source starting
12/06/19 15:32:19 INFO source.NetcatSource: Created serverSocket:sun.nio.ch.ServerSocketChannelImpl[/127.0.0.1:44444]
12/06/19 15:32:34 INFO sink.LoggerSink: Event: { headers:{} body: 48 65 6C 6C 6F 20 77 6F 72 6C 64 21 0D Hello world!. }
Congratulations – you’ve successfully configured and deployed a Flume agent!
Installing third-party plugins
Flume has a fully plugin-based architecture. While Flume ships with many out-of-the-box sources, channels, sinks, serializers, and the like, many implementations exist which ship separately from Flume.
While it has always been possible to include custom Flume components by adding their jars to the FLUME_CLASSPATH variable in the flume-env.sh file, Flume now supports a special directory called plugins.d which automatically picks up plugins that are packaged in a specific format. This allows for easier management of plugin packaging issues as well as simpler debugging and troubleshooting of several classes of issues, especially library dependency conflicts.
Data Ingestion
Flume supports a number of mechanisms to ingest data from external sources.
RPC – An Avro client included in the Flume distribution can send a given file to Flume Avro source using avro RPC mechanism:
$ bin/flume-ng avro-client -H localhost -p 41414 -F /usr/logs/log.10
The above command will send the contents of /usr/logs/log.10 to the Flume source listening on that ports.
Executing commands – There’s an exec source that executes a given command and consumes the output. A single ‘line’ of output ie. text followed by carriage return (‘\r’) or line feed (‘\n’) or both together.
Flume does not support tail as a source. One can wrap the tail command in an exec source to stream the file.
Network streams – Flume supports the following mechanisms to read data from popular log stream types, such as Avro, Thrift, Syslog and Netcat.
Setting multi-agent flow
Two agents communicating over Avro RPC
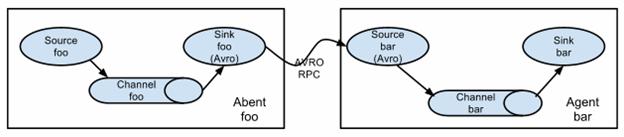
In order to flow the data across multiple agents or hops, the sink of the previous agent and source of the current hop need to be avro type with the sink pointing to the hostname (or IP address) and port of the source.
Consolidation
A very common scenario in log collection is a large number of log producing clients sending data to a few consumer agents that are attached to the storage subsystem. For example, logs collected from hundreds of web servers sent to a dozen of agents that write to HDFS cluster.
A fan-in flow using Avro RPC to consolidate events in one place
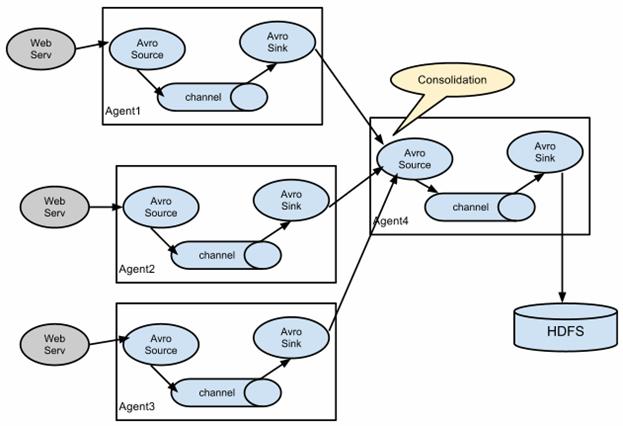
This can be achieved in Flume by configuring a number of first tier agents with an avro sink, all pointing to an avro source of single agent (Again you could use the thrift sources/sinks/clients in such a scenario). This source on the second tier agent consolidates the received events into a single channel which is consumed by a sink to its final destination.
Configuration
Flume agent configuration is read from a file that resembles a Java property file format with hierarchical property settings.
Defining the flow – To define the flow within a single agent, you need to link the sources and sinks via a channel. You need to list the sources, sinks and channels for the given agent, and then point the source and sink to a channel. A source instance can specify multiple channels, but a sink instance can only specify one channel. The format is as follows:
# list the sources, sinks and channels for the agent
<Agent>.sources = <Source>
<Agent>.sinks = <Sink>
<Agent>.channels = <Channel1> <Channel2>
# set channel for source
<Agent>.sources.<Source>.channels = <Channel1> <Channel2> …
# set channel for sink
<Agent>.sinks.<Sink>.channel = <Channel1>
For example, an agent named agent_foo is reading data from an external avro client and sending it to HDFS via a memory channel. The config file weblog.config could look like:
# list the sources, sinks and channels for the agent
agent_foo.sources = avro-appserver-src-1
agent_foo.sinks = hdfs-sink-1
agent_foo.channels = mem-channel-1
# set channel for source
agent_foo.sources.avro-appserver-src-1.channels = mem-channel-1
# set channel for sink
agent_foo.sinks.hdfs-sink-1.channel = mem-channel-1
This will make the events flow from avro-AppSrv-source to hdfs-Cluster1-sink through the memory channel mem-channel-1. When the agent is started with the weblog.config as its config file, it will instantiate that flow.
Configuring individual components – After defining the flow, you need to set properties of each source, sink and channel. This is done in the same hierarchical namespace fashion where you set the component type and other values for the properties specific to each component:
# properties for sources
<Agent>.sources.<Source>.<someProperty> = <someValue>
# properties for channels
<Agent>.channel.<Channel>.<someProperty> = <someValue>
# properties for sinks
<Agent>.sources.<Sink>.<someProperty> = <someValue>
The property “type” needs to be set for each component for Flume to understand what kind of object it needs to be. Each source, sink and channel type has its own set of properties required for it to function as intended. All those need to be set as needed. In the previous example, we have a flow from avro-AppSrv-source to hdfs-Cluster1-sink through the memory channel mem-channel-1. Here’s an example that shows configuration of each of those components:
agent_foo.sources = avro-AppSrv-source
agent_foo.sinks = hdfs-Cluster1-sink
agent_foo.channels = mem-channel-1
# set channel for sources, sinks
# properties of avro-AppSrv-source
agent_foo.sources.avro-AppSrv-source.type = avro
agent_foo.sources.avro-AppSrv-source.bind = localhost
agent_foo.sources.avro-AppSrv-source.port = 10000
# properties of mem-channel-1
agent_foo.channels.mem-channel-1.type = memory
agent_foo.channels.mem-channel-1.capacity = 1000
agent_foo.channels.mem-channel-1.transactionCapacity = 100
# properties of hdfs-Cluster1-sink
agent_foo.sinks.hdfs-Cluster1-sink.type = hdfs
agent_foo.sinks.hdfs-Cluster1-sink.hdfs.path = hdfs://namenode/flume/webdata
#…
Adding multiple flows in an agent – A single Flume agent can contain several independent flows. You can list multiple sources, sinks and channels in a config. These components can be linked to form multiple flows:
# list the sources, sinks and channels for the agent
<Agent>.sources = <Source1> <Source2>
<Agent>.sinks = <Sink1> <Sink2>
<Agent>.channels = <Channel1> <Channel2>
Then you can link the sources and sinks to their corresponding channels (for sources) of channel (for sinks) to setup two different flows. For example, if you need to setup two flows in an agent, one going from an external avro client to external HDFS and another from output of a tail to avro sink, then here’s a config to do that:
# list the sources, sinks and channels in the agent
agent_foo.sources = avro-AppSrv-source1 exec-tail-source2
agent_foo.sinks = hdfs-Cluster1-sink1 avro-forward-sink2
agent_foo.channels = mem-channel-1 file-channel-2
# flow #1 configuration
agent_foo.sources.avro-AppSrv-source1.channels = mem-channel-1
agent_foo.sinks.hdfs-Cluster1-sink1.channel = mem-channel-1
# flow #2 configuration
agent_foo.sources.exec-tail-source2.channels = file-channel-2
agent_foo.sinks.avro-forward-sink2.channel = file-channel-2
Exec Source
Exec source runs a given Unix command on start-up and expects that process to continuously produce data on standard out (stderr is simply discarded, unless property logStdErr is set to true). If the process exits for any reason, the source also exits and will produce no further data. This means configurations such as cat [named pipe] or tail -F [file] are going to produce the desired results where as date will probably not – the former two commands produce streams of data where as the latter produces a single event and exits.
Property Name | Default | Description |
channels | – | |
type | – | The component type name, needs to be exec |
command | – | The command to execute |
shell | – | A shell invocation used to run the command. e.g. /bin/sh -c. Required only for commands relying on shell features like wildcards, back ticks, pipes etc. |
restartThrottle | 10000 | Amount of time (in millis) to wait before attempting a restart |
restart | false | Whether the executed cmd should be restarted if it dies |
logStdErr | false | Whether the command’s stderr should be logged |
batchSize | 20 | The max number of lines to read and send to the channel at a time |
batchTimeout | 3000 | Amount of time (in milliseconds) to wait, if the buffer size was not reached, before data is pushed downstream |
selector.type | replicating | replicating or multiplexing |
selector.* | Depends on the selector.type value | |
interceptors | – | Space-separated list of interceptors |
interceptors.* |
Example for agent named a1:
a1.sources = r1
a1.channels = c1
a1.sources.r1.type = exec
a1.sources.r1.command = tail -F /var/log/secure
a1.sources.r1.channels = c1
The ‘shell’ config is used to invoke the ‘command’ through a command shell (such as Bash or Powershell). The ‘command’ is passed as an argument to ‘shell’ for execution. This allows the ‘command’ to use features from the shell such as wildcards, back ticks, pipes, loops, conditionals etc. In the absence of the ‘shell’ config, the ‘command’ will be invoked directly. Common values for ‘shell’ : ‘/bin/sh -c’, ‘/bin/ksh -c’, ‘cmd /c’, ‘powershell -Command’, etc.
a1.sources.tailsource-1.type = exec
a1.sources.tailsource-1.shell = /bin/bash -c
a1.sources.tailsource-1.command = for i in /path/*.txt; do cat $i; done
JMS Source
JMS Source reads messages from a JMS destination such as a queue or topic. Being a JMS application it should work with any JMS provider but has only been tested with ActiveMQ. The JMS source provides configurable batch size, message selector, user/pass, and message to flume event converter. Note that the vendor provided JMS jars should be included in the Flume classpath using plugins.d directory (preferred), –classpath on command line, or via FLUME_CLASSPATH variable in flume-env.sh.
Property Name | Default | Description |
channels | – | |
type | – | The component type name, needs to be jms |
initialContextFactory | – | Inital Context Factory, e.g: org.apache.activemq.jndi.ActiveMQInitialContextFactory |
connectionFactory | – | The JNDI name the connection factory shoulld appear as |
providerURL | – | The JMS provider URL |
destinationName | – | Destination name |
destinationType | – | Destination type (queue or topic) |
messageSelector | – | Message selector to use when creating the consumer |
userName | – | Username for the destination/provider |
passwordFile | – | File containing the password for the destination/provider |
batchSize | 100 | Number of messages to consume in one batch |
converter.type | DEFAULT | Class to use to convert messages to flume events. |
converter.* | – | Converter properties. |
converter.charset | UTF-8 | Default converter only. Charset to use when converting JMS TextMessages to byte arrays. |