A MapReduce program is composed of four program components
- Mapper
- Partitioner
- Combiner
- Reducer
These components execute in a distributed environment in multiple JVM’s. The two JVMs important to a MapReduce developer are the JVM which executes a Mapper and that which executes the Reducer instance. Every component you develop will execute in one of these JVMs. Unless you have turned on JVM reuse, which is not recommended, each Mapper and Reducer instance will execute in its own JVM. The diagram below shows the various components and the nodes in which they execute.
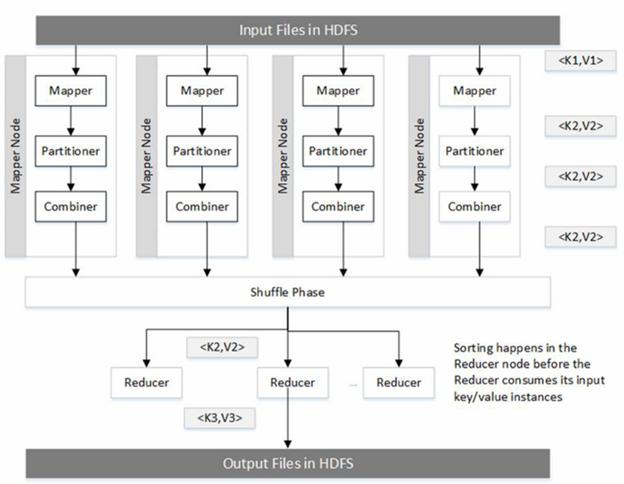
The Mapper, Partitioner and the Combiner all execute on the Mapper node in a single JVM designated for the Mapper. This has many implications. Static variables set in one component can be accessed by other components. If you like using the Spring API, you can exchange messages by configuring a set of objects using dependency injection. As we will discuss soon, these components will execute simultaneously during certain periods of time along with other framework threads which will be launched for various house-keeping tasks.
The goal of Mapper instance, along with the corresponding Partitioner and Combiner instances, is to produce partitions (files), one per Reducer.
A MapReduce program has two main phases
- Map
- Reduce
The Map Phase
As a developer they key interaction you will have with the Hadoop framework is when you make the context.write(…) invocation. See an example invocation from a sample WordCountMapper used in the
public static class WordCountMapper
extends Mapper<LongWritable, Text, Text, IntWritable> {
public void map(LongWritable key, Text value, Context context) throws IOException,
InterruptedException {
String w = value.toString();
context.write(new Text(w), new IntWritable(1));
}
}
This is the invocation where the key and value pairs emitted by the Mapper are sent to the Reducer instance, which is on a separate JVM, and typically on a separate node. In this section, we will review what happens behind the scenes when you make this call. Figure below shows what happens on the Mapper node when you invoke this method
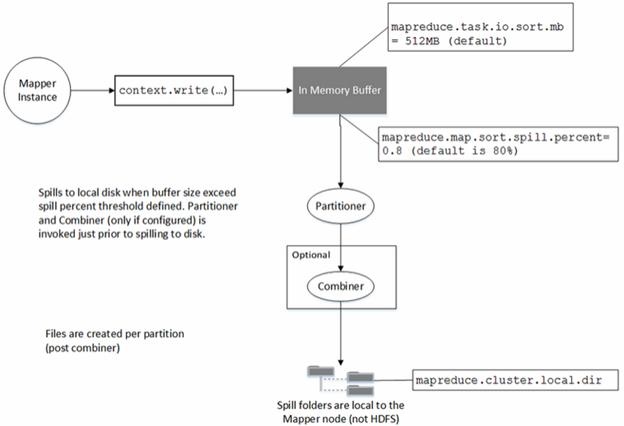
The goal of the Mapper is to produce a partitioned file sorted by the Mapper output keys. The partitioning is with respect to the reducers the keys are meant to be processed by. After the Mapper emits its key and value pair, they are fed to a Partitioner instance that runs in the same JVM as the Mapper. The Partitioner partitions the Mapper output, based on the number of Reducers and any custom partitioning logic. The results are then sorted by the Mapper output key.
At this point, the Combiner is invoked (if a Combiner is configured for the job) on the sorted output. Note that the Combiner is invoked after the Partitioner in the same JVM as the Mapper.
Finally this partitioned, sorted and combined output is spilled to the disk. Optionally the Mapper intermediate outputs can be compressed. Compression reduces the I/O as these Mapper output files are written the disk on the Mapper node. Compression also reduces the network I/O as these compressed files are transferred to the reducer nodes.
The following key events take place on the Mapper node
- The records collected on the Mapper via the context.write invocation fill up a buffer maintained on the Mapper. The size of this buffer is defined by the property mapreduce.task.io.sort.mb. Its default value is 512 MB. The size of this buffer controls the efficiency of the sort process on the Mapper node. Higher the size more efficient the sort. However, memory is a limited resource and increasing this size will limit the number of mappers you can start on a node. Thus there is a trade-off between efficient sorting and the degree of parallelism that can be achieved.
- When the buffer fills up to the extent defined by the property mapreduce.map.sort.spill.percent, a background thread will start spilling the contents to the disk. The default value for this property is 0.8 (80%). The Mapper side invocation of context.write will not block even if this threshold is exceeded if the spill is in progress. Thus the amount of data written to the disk in one spill might exceed this threshold. The spills are made to a file in the directories defined by the property mapreduce.cluster.local.dir in a round robin fashion.
- Before data is written to the disk, the Partitioner is invoked and partitions are created per Reducer. Within each partition, an in-memory sort is performed by key (remember that the Reducer receives its keys in a sorted order. If a Combiner is defined it will be executed on the output of the sort. The mapreduce.map.sort.spill.percent might be exceeded multiple times during the Mapper execution. A new spill file is created each time this threshold is exceeded. When the Mapper finally completes there may be several partitioned spill files on the local disk of the Mapper. They are merged into a single partitioned, sorted file. The number of spills to be merged in one pass is defined by the property mapreduce.task.io.sort.factor. Its default value is 10. If there are more files to merge than defined by the property mapreduce.map.combine.minspills, the Combiner is invoked again before the final file is created. Remember, that Combiner can be invoked multiple times without affecting the final result.
The Reduce Phase
The three main sub phases in the Reduce phase are-
- Shuffle
- Merge/Sort
- Invocation of the reduce() method.
The Shuffle phase – The Shuffle phase ensures that the partitions reach the appropriate Reducers. The Shuffle phase is a component of the Reduce phase. During the Shuffle phase, each Reducer uses the HTTP protocol to retrieve its own partition from the Mapper nodes. Each Reducer uses five threads by default to pull its own partitions from the Mapper nodes defined by the property mapreduce.reduce.shuffle.parallelcopies.
But how do the Reducer’s know which nodes to query to get their partitions? This happens through the Application Master. As each Mapper instance completes, it notifies the Application Master about the partitions it produced during its run. Each Reducer periodically queries the Application Master for Mapper hosts until it has received a final list of nodes hosting its partitions.
The reduce phase begins when the fraction of the total mapper instances completed exceeds the value set in the property mapreduce.job.reduce.slowstart.completedmaps. This does not mean that the reduce() invocations can begin. Only the partition download from the Mapper nodes to the Reducer nodes can initiate.
Merge/Sort – At this point the Reducer has received all the partitions it needs to process by downloading them from the Mapper nodes using the HTTP protocol. The key-value pairs received from the Mapper needs to be sorted by the Mapper output key (or reducer input key).
Each of the partition downloaded from the Mapper are already sorted by the Mapper output key. But all the partitions received from all the Mapper’s need to be sorted by the Mapper output key. This is the Merge/Sort phase. The end result of this phase will be that all the records meant to be processed by the Reducer will be sorted by the Mapper output key (or Reducer input key). Only when this phase completes we are ready to make the reduce() call.
Invocation of the reduce() method – MapReduce developers often wonder why we have the sort phase. The reduce method in the Reducer handles all the values for one key in a single reduce method invocation.
public static class WordCountReducer extends Reducer<Text, IntWritable, Text, IntWritable> {
public void reduce(Text key, Iterable<IntWritable> values, Context context) throws IOException, InterruptedException {
int sum = 0;
for (IntWritable val : values) {
sum += val.get();
}
context.write(key, new IntWritable(sum));
}
}
In the interface to the reduce call, the values are received in the Iterable instance. But are all values for a given key held in memory during the execute of a reduce() call. No! This would easily overwhelm the JVM memory when one key has millions of values. In fact the entire Iterable interface is just that – an interface. In reality the framework is simply iterating the file comprising of Reducer input key-value pairs sorted by reducer key.
When a new key is encountered a new reduce call is made. During the iteration of each value instance from the values instance the value instance is updated with a new set of values (the main reason why you should not rely on references to an earlier value in the iteration). If the iteration of the values instance is skipped or breaks, the framework ensures that the pointer is at the next key in the partition file being processed by the reducer. This is the file that is produced by the “Merge/Sort” process described earlier. It is the sort phase which allows millions of values to be processed efficiently (in one pass while reading a file whose records are sorted by the Reducer input key) for a given key inside the reduce invocation through the familiar Iterable interface without running out of memory or having to make multiple passes on a reducer input file.
Optimizing the Sort/Merge phase on the Reducer node
Some of the parameters which can be utilized to optimize the sort behavior on the Reducer node are
- reduce.shuffle.input.buffer.percent – This is the proportion of heap memory dedicated to storing map outputs retrieved from the mapper during the shuffle phase. If a specific mapper output is small enough it is retrieved into this memory or else it is persisted to the local disk of the reducer
- reduce.shuffle.merge.percent – This is the usage threshold of the heap memory defined by the
- reduce.shuffle.input.buffer.percent at which an in-memory
- merge of the mapper outputs maintained in memory will be initiated.
- reduce.merge.inmem.threshold – This is the threshold in terms of the number of mapper outputs accumulated in memory after which the in-memory merge process initiates. Either the threshold defined by this property or the property defined above (mapreduce.reduce.shuffle.merge.percent) needs be reached for in-memory merge process to be initiated.
Custom Shuffle and Sort – The pluggable shuffle and pluggable sort capabilities allow replacing the built in shuffle and sort logic with alternate implementations. Example use cases for this are: using a different application protocol other than HTTP such as RDMA for shuffling data from the Map nodes to the Reducer nodes; or replacing the sort logic with custom algorithms that enable Hash aggregation and Limit-N query.
A custom shuffle implementation requires a
org.apache.hadoop.yarn.server.nodemanager.containermanager.AuxServices.AuxiliaryService implementation class running in the NodeManagers and a
org.apache.hadoop.mapred.ShuffleConsumerPlugin implementation class running in the Reducer tasks.
The default implementations provided by Hadoop can be used as references:
- apache.hadoop.mapred.ShuffleHandler
- apache.hadoop.mapreduce.task.reduce.Shuffle
A custom sort implementation requires a org.apache.hadoop.mapred.MapOutputCollector implementation class running in the Mapper tasks and (optionally, depending on the sort implementation) a org.apache.hadoop.mapred.ShuffleConsumerPlugin implementation class running in the Reducer tasks.
The default implementations provided by Hadoop can be used as references:
- apache.hadoop.mapred.MapTask$MapOutputBuffer
- apache.hadoop.mapreduce.task.reduce.Shuffle
Encrypted Shuffle – The Encrypted Shuffle capability allows encryption of the MapReduce shuffle using HTTPS and with optional client authentication (also known as bi-directional HTTPS, or HTTPS with client certificates). It comprises:
- A Hadoop configuration setting for toggling the shuffle between HTTP and HTTPS.
- A Hadoop configuration settings for specifying the keystore and truststore properties (location, type, passwords) used by the shuffle service and the reducers tasks fetching shuffle data.
- A way to re-load truststores across the cluster (when a node is added or removed).